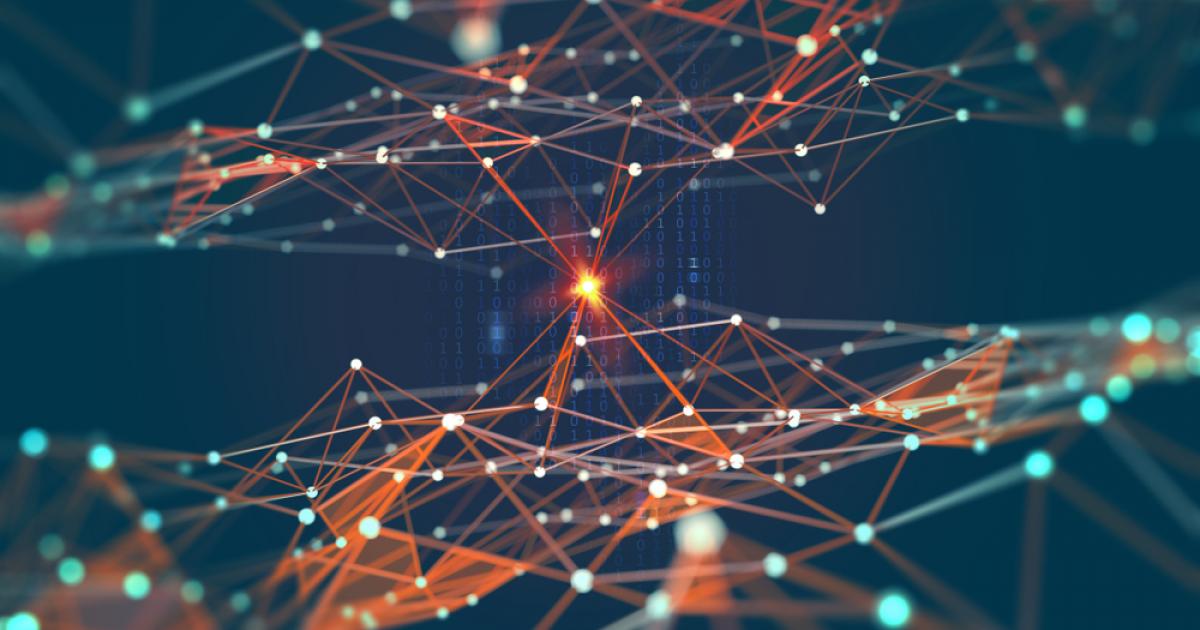
Research background
The analysis of complex systems in terms of networks has recently become an important interdisciplinary topic, which provides a modern tool to study spatio-temporal data. Complex networks often have irregular topological structures, therefore, the statistical analysis of the complex wiring architecture reveals the underlying principles, which initiates a revival of network modeling, developing new models to mimic its genuine properties.
As systems, we currently focus on the reconstruction of climate networks by means of nonlinear time series analysis approaches, working closely with scientists from research domain I. We intend to provide a new approach to study the impact of extreme events such as El Niños, Monsoons or volcanic eruptions on the topology of climate networks, which will allow new insights into the stability of the climate system. Our method may also be valuable to illuminate differences in different climate states of earth's history, e.g. holocene, glacial and cretaceous, and to assess the impact of global warming on the stability of the climate system from a different perspective.
On the other hand, a very important issue in the study of complex systems is the interplay between structure and dynamics. Much attention has been devoted to study the emergence of collective dynamics in complex networks from the viewpoint of relating the propensity for dynamics on a network to the topology and local properties. In particular, one aspect of this interplay is synchronization. Synchronization of oscillators acting on the nodes is one of the widely studied dynamical behavior on complex networks. It has been shown that dynamical processes, like network synchronization, are strongly influenced by the structure of the topology of the underlying network.
Furthermore, in many realistic systems, the feedback of dynamics can reshape the network structures. In this regard, one needs to consider evolving networks under the external influences, for instances, the effect of noise on the system dynamics. In particular, we established collaborations with research domains 2 and 3 to study the complex dynamics of ecological and socio-economic systems. We plan to simulate scenarios for a climate change (global warming, changing water supply, increased weather extreme events etc.) and the impact of different human activities (e.g. fragmentation of habitats) by means of the complex networks' approaches.
Highlights
- Original combination of complex systems science with machine learning techniques
- sub-seasonal complex network-based prediction of extreme climate events
Research Highlights
Extreme Climate Events - Climate Networks
Our work focuses on improving predictability of extreme climatic events and related extreme climate phenomena by studying the complex network representations of various meteorological variables such as temperature, pressure, precipitation and wind obtained from both observational and model data. Climate networks encode the pairwise interactions between the various components of the Earth's climate system. Analysis of the network representation of the different meteorological variables helps to identify the most important regions of climate variability, which is a crucial step to construct prediction schemes. A topological investigation using the recently developed techniques of event synchronization, momentary information transfer and clustering, helps to reveal the underlying spatio-temporal patterns of the climate system. Furthermore, we incorporate unsupervised graph clustering (e.g. community detection) on top of the network structure to uncover the regional sub-components of a larger weather system on different time-scales. The interactions within and between these subsystems provide insights for their representations in climate circulation models, and consequently, facilitate the prediction of extreme events. We finally aim to generalize our methodologies to multivariate data in order to reconstruct multi-layer and interacting networks in order to explore the predictability of climate regime changes on the sub-seasonal scale.
Comparison of degree [(a) and (d)], mean geographical distance [(b) and (e)] and local clustering coefficient [(c) and (f)] elds before and during Very Severe Tropical Cyclone Gaja (Nov 10-19, 2018). Figures in a given column have the same colour scale. (a)-(c) shows the network measures before the cyclone for the period Oct 29-Nov 7, 2018. (d)-(f) gives the network measures for the period Nov 10-19, 2018 during the cyclone. The TC tracks are represented by solid black circles whose sizes are scaled according to the cyclone intensity (DOI: 10.21203/rs.3.rs-282298/v1)
Machine Learning
When it comes to the prediction of extreme events, machine learning methods are usually challenged by the uncertainty and limitation of available data, the difficulty of labeling an extreme event, and the high complexity of the considered system. Instead, complex networks, as an effective approach, could well extract information from even little raw data and successfully capture the signal of some extreme events, such as El Niño events. In tandem with the recent development on neural networks, we aim to fill the gap of the difficulty regarding machine learning methods on the prediction of extreme events by combining network approaches and graph neural networks – a type of neural networks that could cope with graph-structure data – which can always better characterize a network compared to attribute-based approaches.
In particular, to overcome the first and the second problems, we choose to study the network percolation which is highly associated with the network structure. We have successfully developed the state-of-the-art approaches to collapse a given network, which could then be used to label climatic networks. Following that, one of our ongoing topics is the investigation of transfer learning on those climatic networks, aiming at both supervised and unsupervised tasks since climatic networks are usually very difficult to label or only have quite limited labels. To overcome the third problem, our another ongoing topic is the study of advanced machine learning methods on complex time series, such as Autoencoder and Transformer.
Y. Liu, X. Wang and J. Kurths: Optimization of targeted node set in complex networks under percolation and selection, Physical Review E, 98.1, 012313 (2018).
Y. Liu, X. Wang and J. Kurths: Framework of evolutionary algorithm for investigation of influential nodes in complex networks, IEEE Transactions on Evolutionary Computation, 23.6, 1049–1063 (2019).
Y. Liu and J. Kurths: Effects of network robustness on explosive synchronization, Physical Review E, 100.1, 012312 (2019).
J. Fan, J. Meng, Y. Liu, A.A. Saberi, J. Kurths and J. Nagler: Universal gap scaling in percolation, Nature Physics, 16.4, 455–461 (2020).
Tipping Elements and Monsoon Prediction
Another focus of the working group is on predictions of climate phenomena using an innovative methodology combining the Tipping element approach, network approach and machine learning (ML) technique. The methodology is fundamentally different from the numerical weather forecast; it is based on new spatial-temporal regularities (or teleconnection between Tipping Elements) and analysis of observational data.
[1] Surovyatkina E.D., Kravtsov Yu. A. and Kurths Ju., (2005), Phys. Rev. E, 72, 046125
[2] Stolbova V., Surovyatkina E., Bookhagen B., Kurths J. GRL 43, 1–9, 2016
The Tipping elements approach has proven to be successful in forecasting monsoon where other methods failed; it applies to the Indian Summer Monsoon. Combining the tipping elements approach with ML opens new perspectives for creating novel forecasting schemes for events that have not happened yet and regions where forecasts do not yet exist.
We issue a long-term forecast of the onset and withdrawal of the Indian Summer Monsoon (the Southwest Monsoon) for the central part of India - most vulnerable for droughts regions where monsoon forecasts have never been made. The long-term forecast means 40 days in advance for the onset date, and 70 days in advance for the withdrawal date.
The most recent advance in our forecasting Indian summer monsoon has constituted 100% success in central India in the last five years (2016-2020)
We publish every forecast on the PIK Monsoon web page, PIK-News, in Indian newspapers and other media in English and even in the Telugu language for Indian farmers.
African Monsoon forecast
We extended the Tipping Element approach for forecasting the Msimu rains season - the East African Monsoon, over the Southern Highlands of Tanzania. It is a part of the EPICC project. The region of forecast is the grain basket of Tanzania. Our long-term forecasts aid agriculture planning in consolidating resources and strengthening capacity to respond effectively to disasters such as floods and droughts.
The forecast warns farmers (in the Swahili language on the figure) that Msimu rains retreat from southern Tanzania by the second week of May (5-10 May).
The forecasts for Tanzania are distributed to External partners in English and in Swahili languages: Ministry of Health, Department of Emergency, Preparedness, and Response, National Meteorological Authority, the Tanzania Climate Smart Agriculture Alliance (TCSAA), International Crops Research Institute for the Semi-Arid Tropics (ICRISAT).
Predition of Pacific Decadal Oscillation (PDO)
Obtaining an efficient prediction of the Pacific Decadal Oscillation (PDO) phase transition is a worldwide challenge. Here, we employed the climate network analysis to uncover early warning signals prior to a PDO phase transition. This way an examination of cooperative behavior in the PDO region revealed an enhanced signal that propagated from the western Pacific to the northwest coast of North America. The detection of this signal corresponds very well to the time when the upper ocean heat content in the off-equatorial northwestern tropical Pacific reaches a threshold, in which case a PDO phase transition may be expected with the arising of the next El Nino/La Nina event. The objectively detected early warning signal successfully forewarned all the six PDO phase transitions from the 1890–2000, and also underpinned the possible PDO phase transition around 2015, which may be triggered by the strong El Nino event in 2015–2016.
(a) The constructed climate network in the north Pacific. The regions “A” and “B” were determined according to the definition of the PDO. The gray lines show the potential links between nodes in “A” and nodes in “B”. For θ > 0, the link points from “A” to “B”. For θ < 0, the link points from “B” to “A”. If θ = 0, the sea surface temperature anomalies in both nodes vary simultaneously. (b) Relations between the 11-years running mean PDO index (green) and the total degrees (negative links) pointed from “A” to “B”, TDA→B (blue). The dashed line represents the threshold that determines whether the TDA→B decrease is significant. The red/blue vertical bars as well as the triangular symbols on the top of the subfigure mark the El Nino/La Nina events that may trigger the PDO phase transition, following the theory proposed by (Meehl et al., 2016; Meehl & Hu, 2006). PDO, Pacific decadal oscillation (see GRL, https://doi.org/10.1029/2020GL091674).