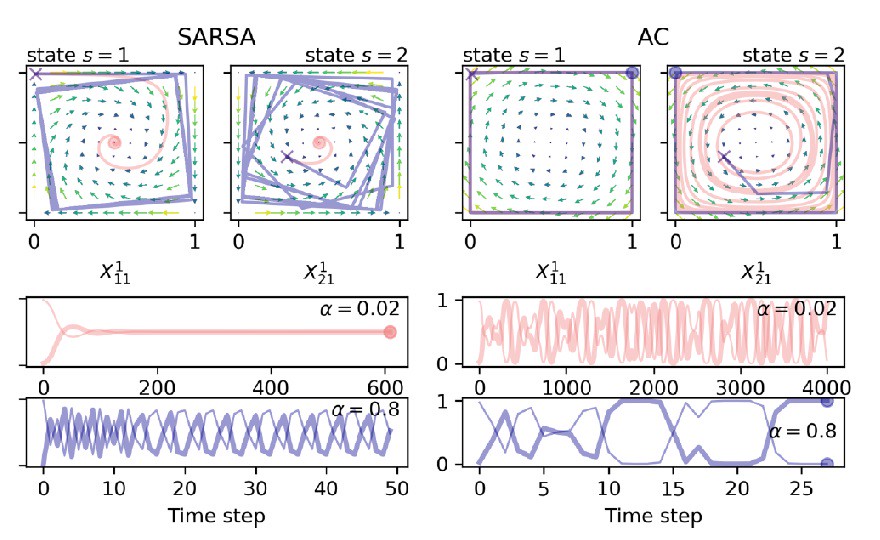
“With our work, we contribute to better understanding what impact artificial intelligence potentially has on society. Using techniques from dynamical systems theory, we find that self-learning agents may not evolve towards a single behaviour. Instead, they may enter a continuous cycle of different behaviours or even evolve on an unpredictable trajectory”, lead author Wolfram Barfuss explains. “Eventually, insights of such dynamical systems studies can be translated back to improve the design of large-scale AI reinforcement learning systems.”
One of the elements distinguishing the study from previous research is the role of the model’s environment. Former research used to limit the variability of the agents’ environment. Yet in reality, environments evolve dynamically, and agents adapt their behaviour accordingly. “We present a methodological extension: By separating the interaction from the adaptation timescale, we obtain the deterministic limit of a general class of reinforcement learning algorithms. This is called temporal difference learning. This form of learning indeed functions in more realistic multistate environments”, says Jürgen Kurths, co-author and chair of the Research Department Complexity Science at the Potsdam Institute.
Artificial intelligence also has great potential for understanding climate impacts. Thus, the Potsdam Institute aims at increasing the use of artificial intelligence in order to assess e.g. how the people potentially react to shocks induced by climate change. This could help to better protect the public from such risks in the future.
Article: Wolfram Barfuss, Jonathan F. Donges, Jürgen Kurths (2019): Deterministic limit of temporal difference reinforcement learning for stochastic games. Phys.Rev. E 99 [DOI: 10.1103/PhysRevE.99.043305]
Weblink to the article: https://journals.aps.org/pre/abstract/10.1103/PhysRevE.99.043305#fulltext